After A.I., welcome A.I.
for Artificial Imagination
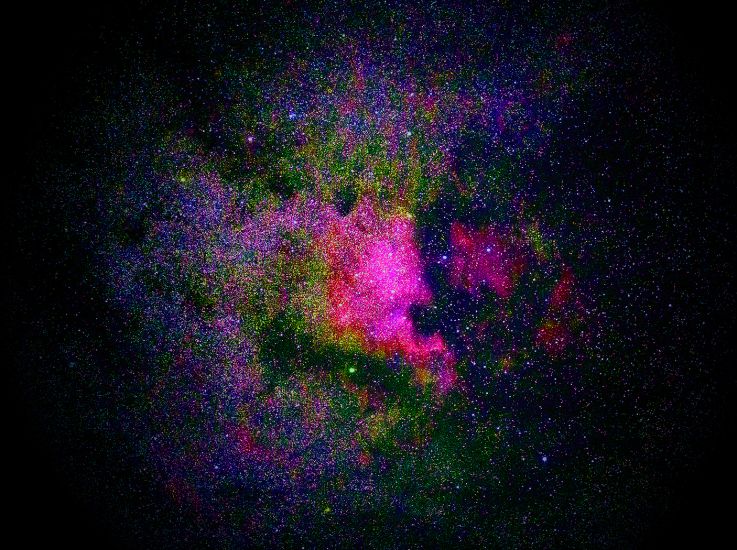
After A.I., welcome A.I. for Artificial Imagination
A few words about the magic inside CogTL’s core, and what makes it a strong companion for teams dealing with risks and opportunities.
Wonderful humans!
Humans are incredible. Humans have been gifted by a body that even the best engineers in the world would not have been able to design. Humans also have the incredible chance to evolve for million years and grow a wonderful brain, able to do so many incredible (and terrible) things.
But it was not enough. As soon as a human was able to use his hands, he began building tools to help himself, to compliment himself. To catch things that he could not catch. To eat more, and to kill before being killed. But even though his primitive needs were fulfilled, he continued inventing things, towards "a better version of himself". He invented locomotion means to move faster. He invented tools to be more efficient. And as science evolved, human invented calculators and computers to "think" faster, soon beating our brain big time at resolving complex calculations.
A better version of ourselves
But already way before computers existed, this dream of "a better version of ourselves" existed, and computer science opened some doors. What is a program else than a big and complex decision tree? Computers soon had the capacity to mimic humans to a certain extent, and we wanted to make them converge towards a human brain. There is no surprise that the term "Artificial Intelligence" came up with John McCarthy in 1955, and that the Turing's test was invented by Alan Turing himself, one of the fathers of today's computer science, during the same decade: the Turing's test goal is precisely aiming at measuring the capacity of a computer to mimic a human's conversation.
Back to more modern computer science: as soon as it became possible, automation and cybernetics made us build machines that represented physically better versions of ourselves: we invented machines that performed the same moves as us, but 24/7. Machines that lifted much more weight than us. Machines that were able to perform very precise moves like us, but in lethal environments for humans - in space, in nuclear power plants, in mine fields...
But a better physical version of ourselves was not enough: we also wanted to be able to reproduce the complex reasoning of the human brain. Artificial Intelligence research fields emerged, and in particular machine learning algorithms. With machine learning, we try to teach a machine how to learn to solve complex problems alone without hardcoding all the rules. That is a bit like raising a child: you don't give a book to your newborn, explaining him or her how to move, how do speak, how to do things and how to behave in society. He or she learns by observing you, by trying and failing, all of this in a very instinctive way. Machine learning algorithms do basically the same, and the outcome is generally a system so much complex that nobody's able to explain how it works, but ... it really mimics human decisions or behavior, but with a much more consistent and constant way than humans.
So ... are we done? Is THAT a better version of ourselves?
Our postulate is that it's far from being sufficient to cover all problems that a human is facing. Our brain is not just a complex "logic" machine. There is a big missing piece in our current virtualized artificial brains: imagination. Imagination is probably the greatest feature of our brain. Imagination is what gives us the power to defeat algorithms by performing unattended actions. Imagination is what makes geniuses and artists. But in our modern businesses, imagination is also the root of risk management: by imagining multiple potential negative scenarios, you get to have a better grip on your risks. On the opposite side, imagination is also the key for market developments. By imagining the consequences of our actions, imagination gives us the ability to grow our businesses in new directions.
This is precisely the key objective of our CogTL engine: to give this ability to our computers to recognize that several scenarios are possible with what you know, to combine them, to combine specific and analytic scenarios with abstract high-level concepts - and finally get a global distribution of your factors of interest (risks, or opportunities) in your data.